Online Learning
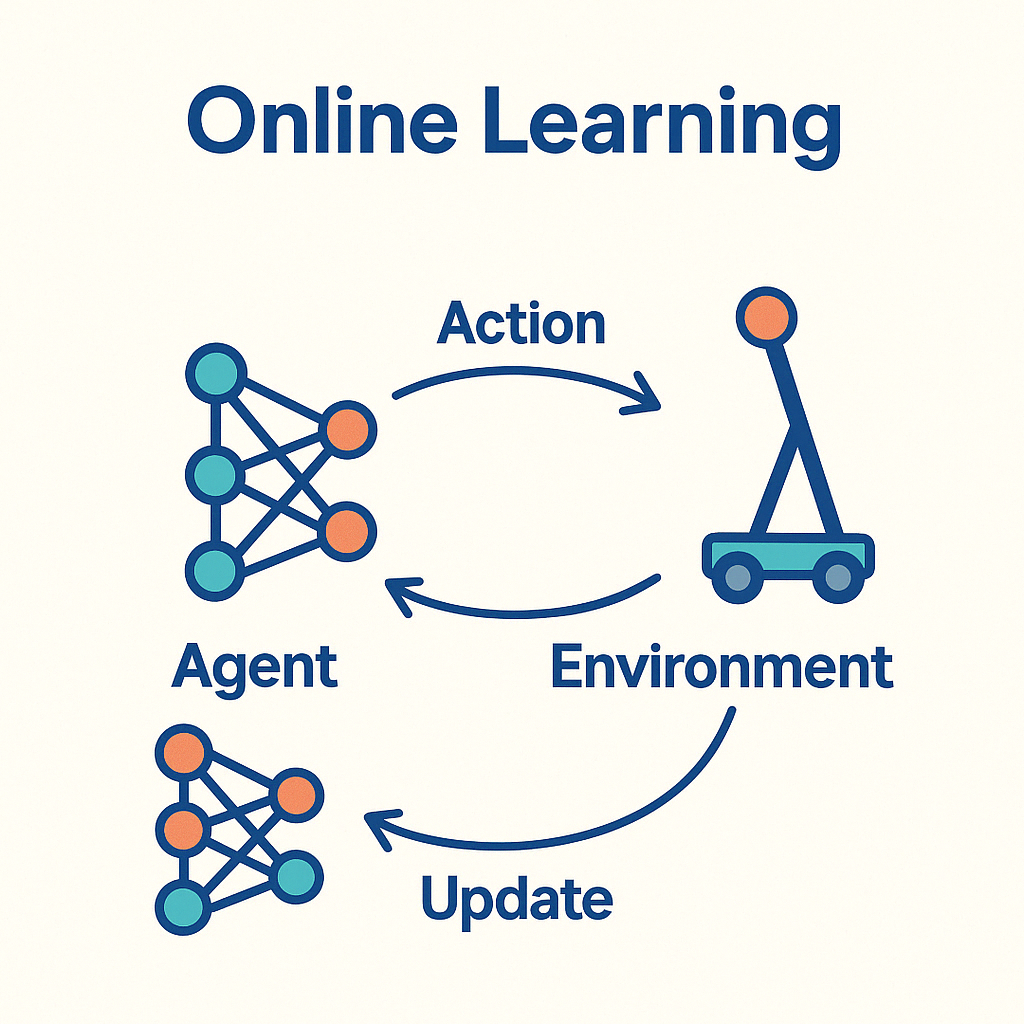
Agent and Environment
In online learning settings, an agent repeatedly interacts with an environment over time. At each time step, the agent takes an action based on the current policy, receives feedback from the environment (such as a cost or reward), and updates its internal parameters accordingly. The environment may be stochastic, adversarial, or even partially observable.
Cost and Update Policy
After each action, the agent receives a cost signal that reflects how suboptimal the action was. Using this cost, the agent performs an online update to its policy or model parameters. This update is typically computed using gradients of the loss function, or more generally, via a regret-minimizing algorithm. The goal is not just to minimize instantaneous cost, but to improve over time with minimal cumulative error.
Regret - Performance Index
A fundamental performance metric in online learning is the regret, which measures the difference between the cumulative cost incurred by the agent and that of the best fixed policy in hindsight. Formally, for time horizon T, the regret is defined as:
Regret(T) = ∑t=1T ct(xt) - minx∈X ∑t=1T ct(x)
where ct
is the cost function at time t and xt
is the decision at time t. Low regret implies that the agent's policy performs nearly as well as the best fixed decision in hindsight.
Online Convex Optimization
Online Convex Optimization (OCO) provides a general framework for online decision-making under convex cost functions. At each round, the agent selects a point in a convex set, receives a convex cost function, and incurs the corresponding cost. The agent's goal is to adaptively minimize regret over time. This framework is powerful because it applies to a wide range of applications including control, finance, and online classification.
Online Gradient Descent
One of the most fundamental algorithms for OCO is Online Gradient Descent (OGD). At each step t, the agent updates its decision xt+1
using the gradient of the current cost function:
xt+1 = ΠX[xt - η∇ct(xt)]
where η
is the learning rate and ΠX
denotes projection onto the feasible set. OGD ensures sublinear regret under mild conditions, making it a powerful tool for real-time learning and control.