Reinforcement Learning
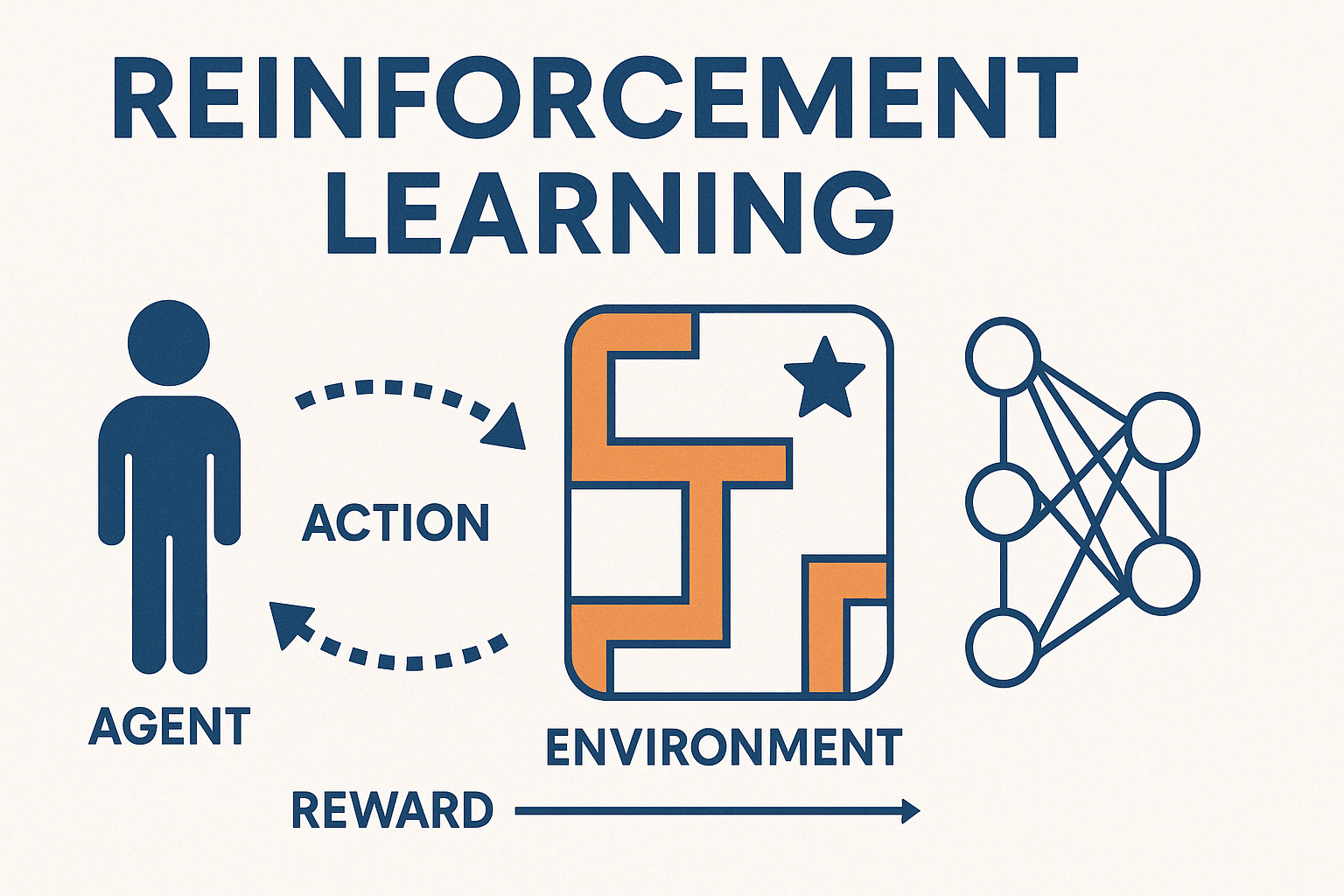
Reinforcement Learning Overview
Reinforcement Learning is a paradigm where an agent learns to make decisions by interacting with an environment to maximize cumulative rewards. An agent observe current state, an agent decides and takes an action and loop these steps. An agent is given a reward function at each time step and update his policy to maximize accumulated rewards.
Compared to Supervised Learning
Machine Learaning
With arise of Machine Learning, various models incredibly perform well in various tasks: image classification, NLP, sounds. It is often assumed sufficient data for training. Meta's Llama3 is a great large language model trained with 15 trillion tokens *1. Lots of large models for image recognition were trained with over 1 million images. However, not all real-world tasks have righ enough labeled data for training. Learning with small data is often difficult; self-supervised learning is for small labeled data and unlabeled data.
RL with Simulator
Reinforcement Learning with a simulator can train a model without data because lots of simulation data can be obtained through a simulation. In addition, Reinforcement Learning with given data such as expert people logs is capable of improving learning efficiency. If small but good data exists, some algorithms have been suggested: Imitation Learning. Furthermore, Reinforcement Learning is also applicable to costy simulation task such as a robot training and a drawn control. At first, a policy is not well skilled and fails many times, which leads to the trouble of them. Therefore, Reinforcement Learning is employed to bring a policy to a sufficiency competent behavior.
References
- Meta AI. Introducing Meta Llama 3